Ph.D. Student @ETH Zürich
Previously @EPFL, @MIT, @Amazon
Working on self-supervised representation learning,
multimodal learning & generative modelling.
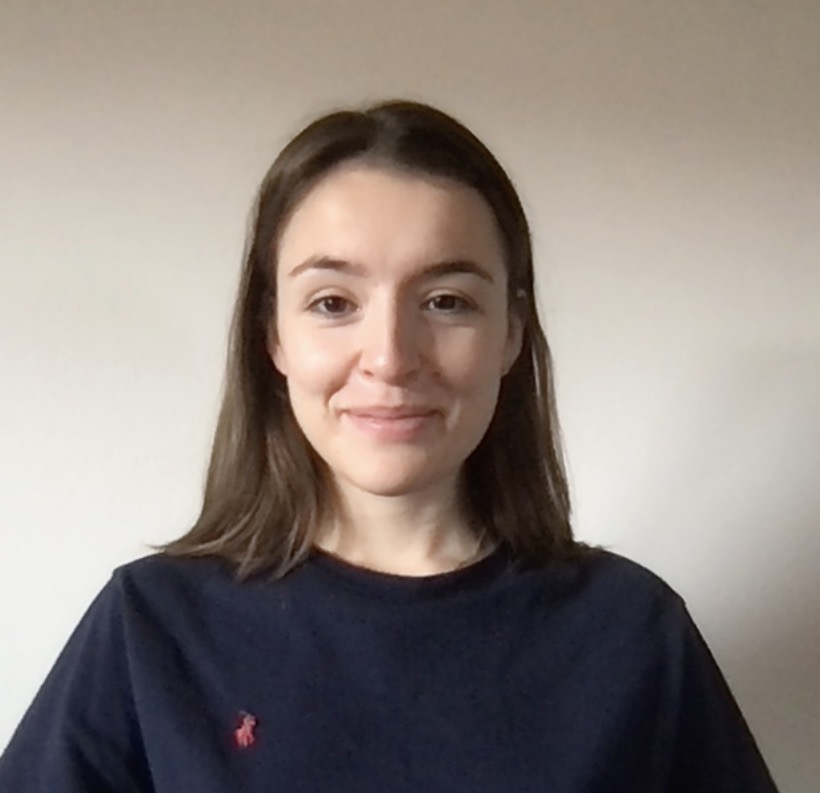
About Me
I am a doctoral student at ETH Zürich and an ETH AI Center fellow, advised by Prof. Julia Vogt and Prof. Bernhard Schölkopf. In April 2025, I started an internship at Apple MLR in Barcelona. In 2024, I interned at Amazon Science in Tübingen, Germany, where I worked on diffusion-based generative modelling. Prior to joining ETH, I graduated from EPFL after a master’s thesis at the MIT under the supervision of Prof. Satrajit Ghosh. At MIT, I worked on the generation of 3D brain scans using GANs.
I am broadly interested in representation learning, (probabilistic) self-supervised (SSL) methods and generative modelling, more specifically understanding how current methods work to then leverage this knowledge to build general representations.
During my PhD, I have been thinking about how we learn representations from observations in unsupervised settings. While discriminative methods (e.g., SimCLR, DINO) generally outperform reconstruction/generative ones (e.g., VAE, MAE), they often rely on less principled approaches. Instead reconstruction-based approach carry their load of challenges, but are driven by principled objectives and require less prior knowledge about the downstream tasks of interests.
Early on during my PhD, I used identifiability and causal representation learning to better understand discriminative SSL in multi-modal settings. Later on, I explored latent variable models as a mean to explain SSL and unify discriminative and generative methods. More recently, I’ve explored Masked Autoencoders and proposed the space of principal components as a more principled and effective masking space for reconstructive SSL. Finally, I am currently looking into the training dynamics of discriminative SSL, specifically how data characteristics affect these dynamics.
More information about me can be found in my resume
Publications
News
Apr 1, 2025 | I started an internship at Apple MLR ! |
---|---|
Jan 22, 2025 | Our paper on Cross Entropy Is All You Need to Invert the Data Generating Process was accepted as an Oral to ICLR 2025! |
Oct 9, 2024 | Our paper on Self-Supervised Learning with Instance Discrimination Learns Identifiable Features was accepted to Neurips Workshops! |
Sep 1, 2024 | Our paper on A Probabilistic Model Behind Self-Supervised Learning was accepted to TMLR! |
Nov 1, 2023 | Joined Amazon for a Research Internship ! |
Mar 1, 2022 | Our paper on Multimodel Contrastive Learning was accepted at ICLR 2023 ! |
Sep 1, 2021 | Joined ETH Zürich as an ETH AI Center Fellow for my Ph.D. |
Mar 1, 2020 | Joined MIT for my Master’s Thesis at the Senseable Intelligence Group. |
Sep 1, 2014 | Joined EPFL for a Bachelor’s & Master’s in Life Sciences Engineering |
Education
Sep 1, 2021 | Ph.D. in Computer Science @ETH Zürich and @ETH AI Center |
---|---|
Feb 1, 2020 | Master’s Thesis @Senseable Intelligence Group, MIT under the supervision of Prof. Satrajit Ghosh. |
Sep 1, 2014 | Bachelor’s & Master’s @EPFL, Faculty of Life Sciences Engineering |
Aug 1, 2014 | Left the Netherlands for Switzerland! |